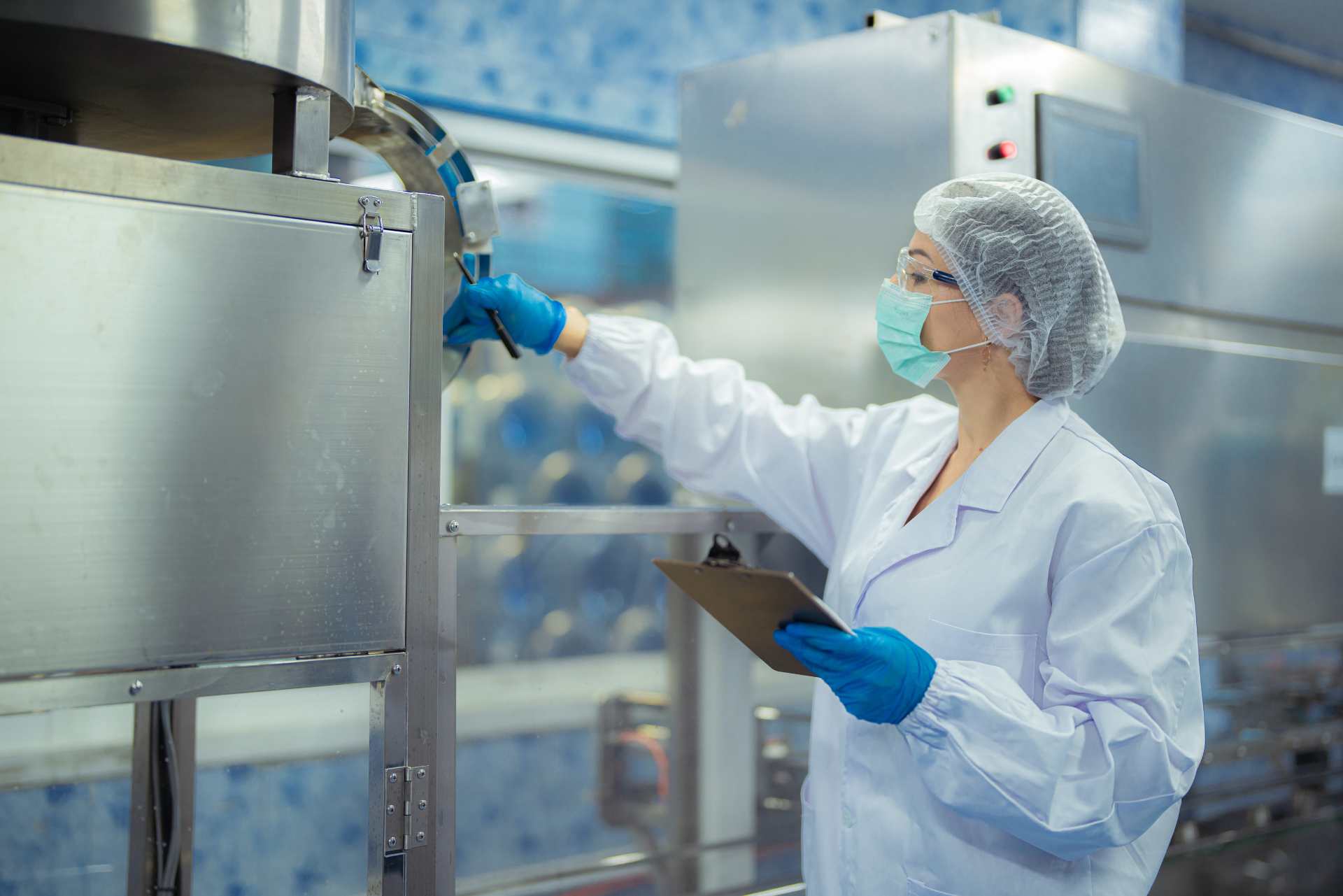
22 Apr Optimising Potency Assays with Design of Experiments (DoE): A Practical Guide for Biologics
Real-world examples of DoE in ADCC, vaccine, and nanobody potency assays.
In the complex and regulated world of biologics, potency assays are among the most critical tools used to ensure the quality, efficacy, and consistency of therapeutic products. Whether it’s for release testing, stability studies, or development milestones, a robust potency assay is essential. Yet, developing one that performs consistently under real-world conditions can be a challenge.
Design of Experiments (DoE) offers a statistically rigorous method to build, refine, and troubleshoot potency assays by understanding the influence of multiple variables simultaneously. It allows scientists to reduce the number of experiments needed while uncovering critical factors and their interactions, which traditional one-factor-at-a-time (OFAT) approaches often miss.
Why Intelligent Potency Assay Design Matters
Potency assays are influenced by a multitude of factors: the number of cells per well, incubation time, reagent concentrations, buffer composition, and more. Most of these factors do not influence the assay in isolation—or in predictable ways.
Using the Quality by Design (QbD) framework, assay developers are encouraged to start with predefined objectives and take a risk-based approach to manage variables throughout the lifecycle. DoE complements QbD by enabling the efficient investigation and optimisation of assay parameters.
Case Study: Rescue of a Drifting ADCC Potency Assay
A real-world example from training illustrates how a validated ADCC potency assay—used to measure the cytotoxic effect of therapeutic antibodies—began to show unacceptable variability in QC. Suspecting three potential sources (plate type, buffer, and the order of reagent addition), the team applied a full factorial DoE with two levels for each factor.
The results were clear: switching to complete growth medium and reversing the order of reagent addition (adding target cells before antibody) significantly improved reproducibility. This reduced CV from 36% to within acceptable limits. The study not only resolved the variability issue but highlighted how DoE can provide evidence-based direction for revalidation.
Case Study: Vaccine Potency Assay Optimisation
In another example, a vaccine potency assay was developed to monitor the ratio of two surface glycoproteins—prM and E—critical to immunogenicity. A screening DoE was used to evaluate which of the many potential parameters (e.g., shaking speed, incubation temperature, antibody concentration) had the most impact on assay performance.
Through risk scoring and fractional factorial design, the team narrowed down the factors that truly mattered. Using JMP software, they created prediction profiler plots, revealing that adjusting parameters like sample volume and incubation temperature could increase the assay’s desirability score to 90%. These optimised conditions were then tested in real assays, confirming improved robustness and precision.
Case Study: Pre-Validation of an Anti-IgE Nanobody Assay
In preparation for preclinical studies, a potency assay was developed to test an anti-IgE nanobody designed to inhibit allergen-induced basophil degranulation. To pre-validate the method, the developers used a full factorial DoE investigating five variables: cell count, IgE concentration, allergen concentration, pre-incubation time, and assay incubation time.
The DoE revealed optimal conditions—such as incubating for exactly one hour—and helped determine the continuous value of factors like cell number for maximum response. When tested under these conditions, the assay passed all validation parameters: whole plate uniformity, specificity, accuracy, precision, and robustness.
DoE vs OFAT: A Strategic Advantage
For potency assays, the stakes are high. OFAT approaches may appear simple, but they are time-consuming and blind to interactions. In contrast, DoE methods—such as fractional factorial and response surface designs—allow scientists to test more variables with fewer runs, gaining insights into main effects, two-way interactions, and even non-linear (quadratic) behaviours.
For example, in a neutralising antibody (NAb) assay measuring caspase-3/7 activity, a fractional factorial DoE was used to investigate six variables including serum concentration, drug amount, and two stages of incubation. While protein G concentration showed no main effect, it had a significant interaction with drug concentration—information an OFAT approach would have completely missed.
Key Considerations: Setting Levels and Statistical Power
Choosing appropriate levels for each factor is critical. Wide ranges may seem more informative but reduce statistical power. Smaller, strategically spaced levels—based on prior knowledge or exploratory data—allow the DoE software to make better predictions with fewer runs.
In one assay, rather than spacing cell counts from 10,000 to 1 million, developers tested 300,000 to 500,000 cells per well. This improved the ability to detect meaningful differences and reduced the overall number of experiments.
Desirability Profiling: Quantifying Success
One of the most powerful features of DoE is the use of desirability functions. These combine multiple assay performance metrics (e.g., EC50, slope, signal range) into a single score between 0 and 1. Using software like JMP, teams can model how changing one factor affects desirability while keeping others constant.
In practice, this means you can visually explore trade-offs and set criteria for what constitutes an optimal assay condition. This was key in identifying final assay setups for both the vaccine and nanobody potency assays.
Conclusion: Smarter Potency Assays with DoE
The development and maintenance of potency assays are critical to biologics success—and Design of Experiments is a transformative tool in that process. From development and troubleshooting to validation and tech transfer, DoE provides:
- Insight into critical variables and their interactions
- Fewer experimental runs with higher informational yield
- Improved assay robustness, reproducibility, and QC friendliness
By embedding DoE into the assay lifecycle, biopharma teams can reduce risk, accelerate timelines, and ensure that their assays—and their products—perform to the highest standards.
Written by Educo Life Sciences Expert, Melody Janssen
Dr. Melody Janssen is an expert in bioanalysis and immunogenicity testing of biopharmaceuticals with in-depth experience as a project manager, trainer and senior expert in CROs and pharma/biotech companies. She worked predominantly on developing and validating ligand-binding and cell-based assays for PK/Tox and immunogenicity studies for biologics, biosimilars and vaccines and her work supported numerous regulatory filings. Dr. Janssen is experienced in the regulatory framework related to bioanalytical method establishment and validation including FDA, EMA, ICH guidelines and USP and Ph.Eur. monographs.
This article was written from an interview and from materials taken from the course, Potency Assay Lifecycle From Development to Validation, QC for Biopharmaceuticals and Cell & Gene Therapies.
Sign up for the Educo Newsletter
Stay up-to-date with the latest free trainer interviews, articles, training courses and more. We will also keep you updated on upcoming courses. Complete the form below.
View Our Range of Training Courses
Discover our range of online and classroom courses covering various topics within Pharmaceuticals (Regulatory Affairs), Biopharmaceuticals, Cell & Gene Therapies and Medical Devices & IVDs.